Predictive Analysis in Insurance: Real-Life Examples Creating Win-Win Scenarios for You and Your Customers
The insurance industry, once considered traditional and resistant to change, is now at the forefront of a digital revolution fueled by analytics. As per a recent study, the worldwide insurance analytics market is experiencing rapid growth with a Compound Annual Growth Rate (CAGR) of 15.1%, poised to achieve a valuation of USD 20.6 billion by the year 2026.
This seismic shift in market size signifies a fundamental transformation in how insurance companies leverage data to make informed decisions. As we stand on the cusp of this data-driven evolution, it is necessary to explore the driving forces behind this exponential growth and understand how analytics is reshaping the future of insurance on a global scale.
Why predictive analytics is important in insurance?
At its core, predictive analytics empowers insurance companies to make data-driven decisions, resulting in more accurate risk assessments, better operational efficiency, and improved customer experiences. It is a key tool for staying competitive and resilient in the insurance industry. But let’s take a closer look at the main points:
Risk assessment
Predictive analytics helps insurance companies assess and predict risks more accurately. By analyzing vast amounts of data, including historical claims data, customer information, and external factors, insurance companies can better understand the likelihood of certain events.
Fraud detection and prevention
The insurance industry is susceptible to fraudulent activities, and predictive analytics can identify and prevent fraud. With the help of examining patterns and anomalies in data, insurers can flag potentially fraudulent claims or actions, avoiding financial losses and maintaining the integrity of the insurance system.
Customer segmentation and personalization
Predictive analytics allows insurers to segment their customer base more effectively. Insurance companies can tailor their products and services by understanding customer behavior, preferences, and needs, creating a more personalized and satisfying experience for policyholders.
Operational efficiency and cost reduction
Insurance companies can use predictive modeling to forecast claims volume, allocate resources more effectively, and identify areas for cost reduction.
Improved customer experience
Predictive analytics allow insurance companies to offer customized recommendations and timely assistance.
How is predictive analytics used in insurance?
The predictive analytics system follows a step-by-step process with 6 main stages. It smoothly guides the insurance industry through collecting data, developing models, assessing risks, processing claims, engaging with customers, and constantly monitoring for ongoing improvements.
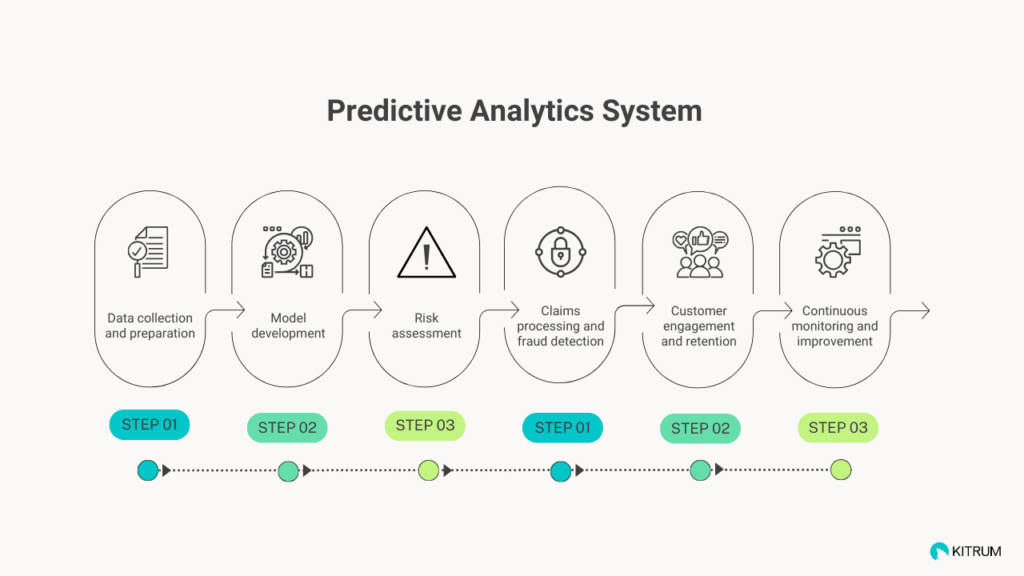
Stage 1: Data сollection and preparation
- Gathering and compiling relevant data from various sources, including historical claims data, customer information, and external data sets.
- Cleansing and preprocessing the data to ensure accuracy and consistency.
- Selecting and creating variables that are most relevant for predictive modeling.
Stage 2: Model development
- Choosing appropriate predictive modeling techniques, such as regression analysis, machine learning algorithms, or neural networks.
- Training the models using historical data, allowing them to learn patterns and relationships.
- Validating and fine-tuning the models to ensure they are accurate and robust.
- Incorporating business rules and considerations into the modeling process.
Stage 3: Risk assessment
- Applying predictive models to assess and predict risks associated with potential policyholders.
- Refining underwriting processes based on model insights, determining appropriate premiums and coverage.
- Tailoring insurance products to individual risk profiles identified through predictive analytics.
Stage 4: Claims processing and fraud detection
- Using predictive models to assess the legitimacy of claims by analyzing historical claims data.
- Flagging potentially fraudulent claims through pattern recognition and anomaly detection.
- Expediting claims processing for legitimate claims, enhancing customer satisfaction.
- Implementing early intervention strategies to prevent or mitigate fraudulent activities.
Stage 5: Customer engagement and retention
- Leveraging customer segmentation derived from predictive analytics to create targeted marketing strategies.
- Developing personalized communication channels based on customer preferences.
- Implementing customer retention strategies, including personalized loyalty programs and cross-selling opportunities.
- Continuously analyzing customer data to enhance overall customer experience.
Stage 6: Continuous monitoring and improvement
- Establishing a feedback loop for continuous monitoring of model performance.
- Regularly updating models with new data to ensure relevance and accuracy.
- Adapting to changes in the insurance landscape, market dynamics, and customer behaviors.
- Implementing improvements and refinements based on ongoing analysis and feedback.
3 predictive analytics use cases in insurance
Adopting predictive analytics, often coupled with increased automation, is a positive development in the insurance industry. According to a survey, 80% of the surveyed companies already employing predictive analytics reported experiencing favorable business outcomes.
AVIVA improves auto-claims
Aviva Canada, a leading property and casualty insurance group, significantly improved its auto claims process to enhance customer experience. Responding to customer feedback about the lengthy and uncertain nature of auto claims settlements, Aviva invested in innovation. In June 2019, the company deployed a Vehicle Remedy Tool, integrating real-time predictive analytics into the auto claim setup process. This tool, seamlessly integrated into Aviva’s ClaimCenter system, provides adjusters with an instant and accurate repair prediction within approximately 5 seconds.
By leveraging data science API internally, Aviva simplified decision-making for adjusters and offered customers certainty about claim outcomes at the first notice of loss. This initiative resulted in a 59% reduction in customer cycle time on total losses, a 52% decrease in rental days, and a 56% decrease in storage days. The tool’s success is evident in improved customer satisfaction, as reflected in significantly enhanced Net Promoter Scores (NPS). Aviva’s commitment to addressing customer concerns and leveraging predictive analytics has not only streamlined its claims process but also exemplifies a customer-centric approach in the insurance industry.
Nirvana raises $57M to bring AI to commercial trucking insurance
Nirvana Insurance aims to revolutionize the insurance industry by leveraging IoT and sensor data to empower fleets. With 90% of fleets having fewer than 50 trucks, Nirvana addresses challenges faced by small businesses in the industry. The company focuses on using data analytics and AI to offer tailored risk management solutions and unlock cost savings for commercial fleets.
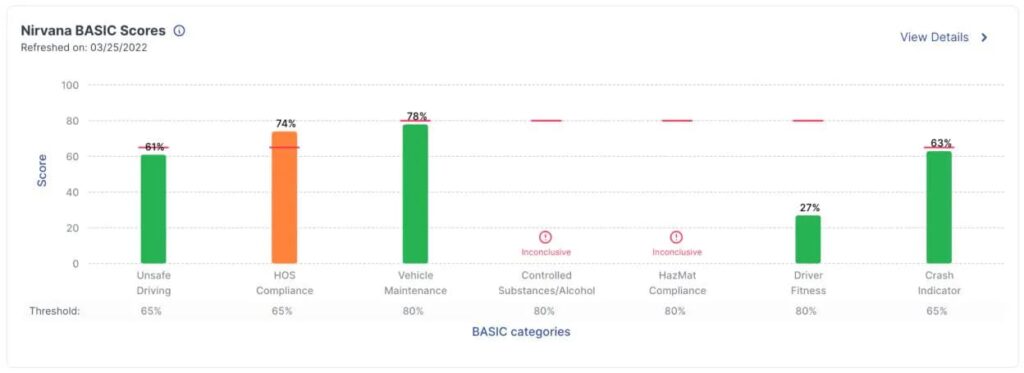
Raviraj Jain, Partner at Lightspeed Venture Partners, commends Nirvana’s pioneering approach, emphasizing the need to move away from traditional, one-size-fits-all insurance models. Targeting the trucking industry, Nirvana leverages truck sensors and billions of data points to create risk models, aiming to provide faster, customized quotes and improved claims processes. The growth of Nirvana is attributed to the vast potential of AI, data analytics, and IoT in Insurance.
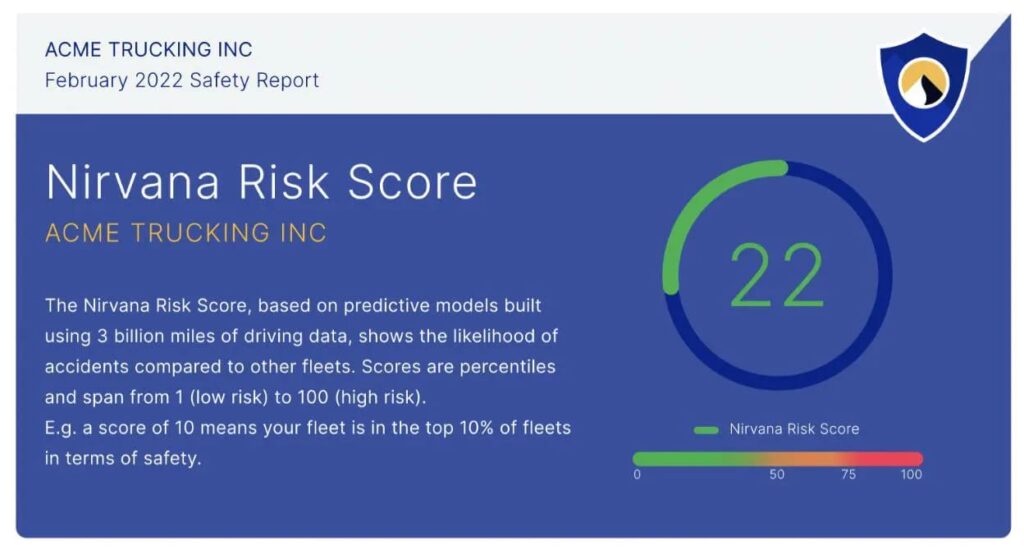
Thus, Nirvana Insurance has secured $57 million in a Series B funding round, doubling its valuation to over $350 million. The platform has witnessed a 30-fold growth since its 2022 launch. The funding will support further platform expansion and business growth.
Alpha Insurance using predictive analytics
Alpha Insurance faced the increasing threat of automobile insurance fraud, which was causing significant financial losses to the industry, amounting to billions. Armed with historical data, the company implemented predictive analytics to combat this issue. With the help of predictive analytics, Alpha Insurance navigated a data-driven journey against automobile insurance fraud. Employing regression, decision trees, neural networks, gradient boosting, and ensemble techniques, they honed in on optimal models. The selection, guided by misclassification rate and ROC metrics, led to a powerful tool. This tool scored new data, exposing potential fraud and empowering Alpha Insurance to cut losses and achieve victory over fraudulent claims.